1
/
of
1
slot online
BIGLUCK8: Situs Slot Online 2024 Deposit Pulsa Telkomsel dan XL Resmi
BIGLUCK8: Situs Slot Online 2024 Deposit Pulsa Telkomsel dan XL Resmi
Banyak Pemberitahuan BIGLUCK8 sebagai Situs Slot Online Indonesia Terpercaya Dengan minimal deposit 5rb dan promosi deposit pulsa telkomsel dan xl tanpa potongan 100%. sebagaiman yang perlu diketahu bahwa kami sebagai Bandar ataupun Agen selalu menyedikan Link paling gacor serta List RTP Live Paling jitu, Terdapat lebih dari 888 permainan BIGLUCK8 Slot Online Resmi dan SLOT88 penyedia Permainan Tanpa potongan atupun bonus terbesar 2024.

Regular price
Rp 10.000,00
Regular price
Rp 25.000,00
Sale price
Rp 10.000,00
Unit price
/
per
Couldn't load pickup availability
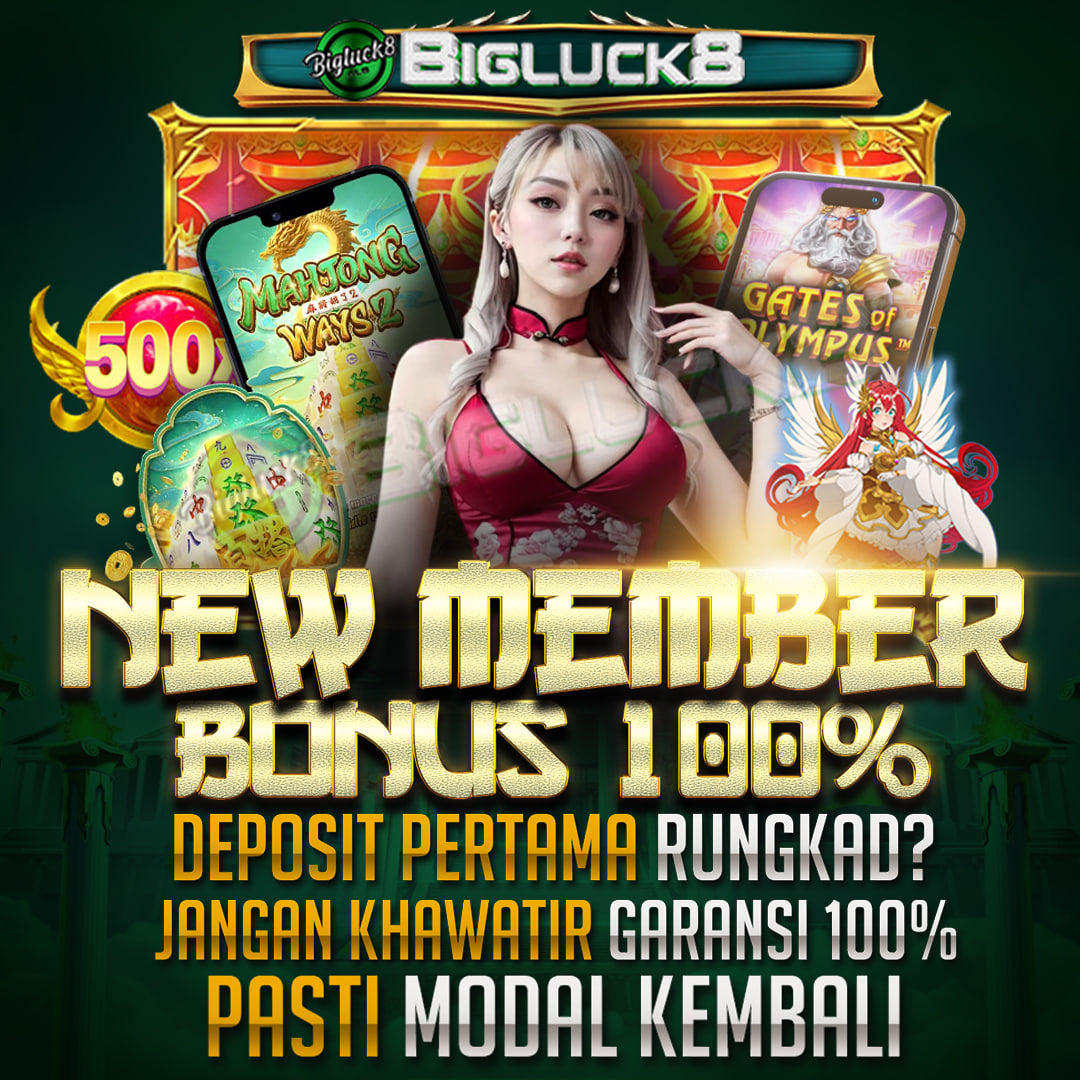